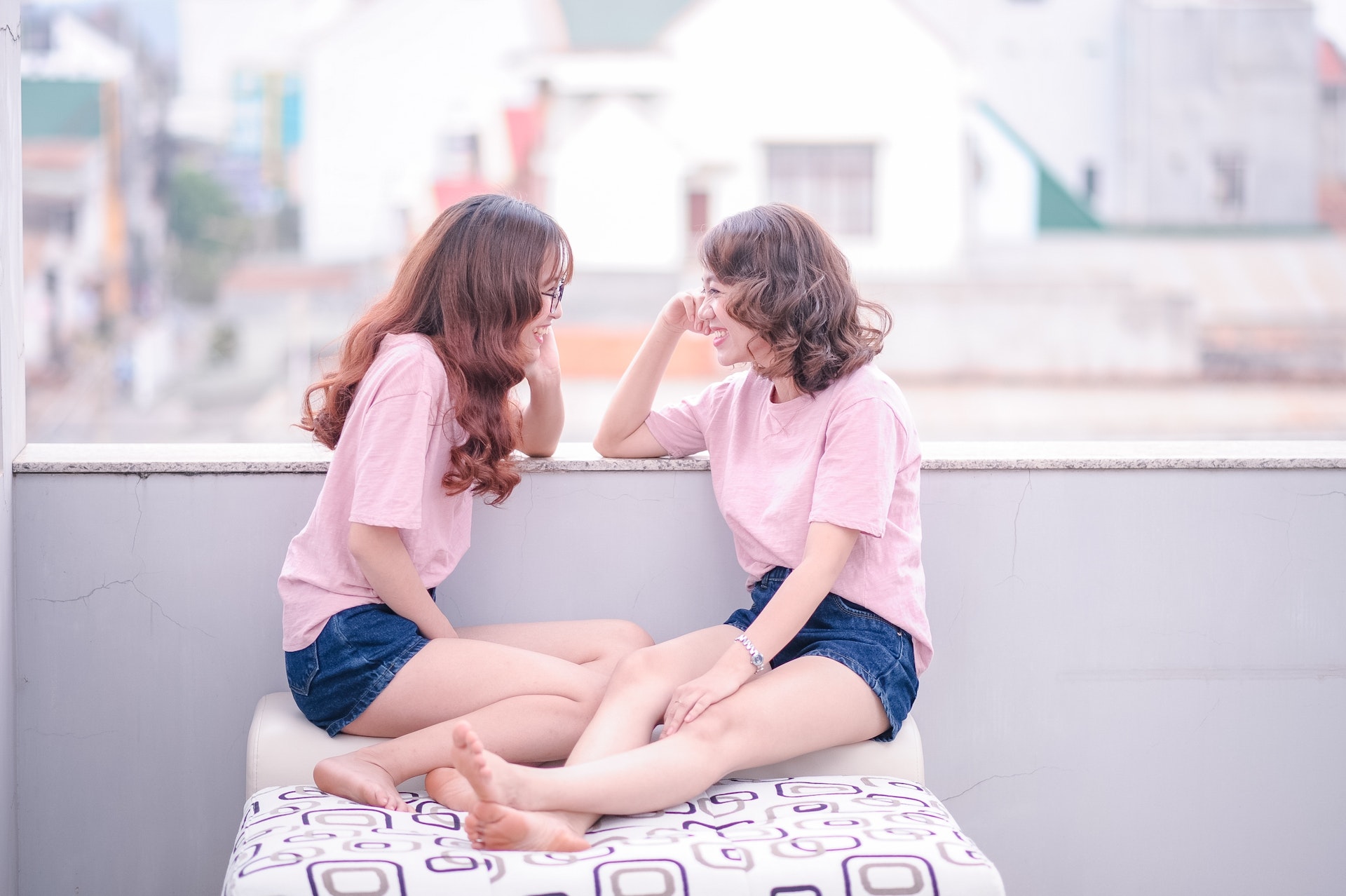
Sir Isaac Newton has famously said, “If I have seen further, it is by standing upon the shoulders of giants.” This phrase is also the basis of research. Apart from being novel and significant, replicability and reproducibility in research is critical for other scholars to be able to build on your work and make new discoveries.
What does reproducibility in science and research mean?
Reproducibility in science and research means that others are able to come up with the same results using the data and methods as defined in the original study. Being able to repeat experiments only to get the same results is seen as validation of the original research, which in turn is considered as a reliable source for future developments in the same or related field of study. Stanford University medical researcher and a leading scholar on the topic of reproducibility in science, John Ioannidis says reproducibility in science is not linked with ensuring the ‘correctness’ of results, it’s an important aspect to ensure transparency of the journey to reaching the conclusions in any research study.1
In the recent past, several published studies have crumbled when subjected to scrutiny.2 This has raised reproducibility in science as an important topic of discussion in the academic community. Without scientific reproducibility and replicability in research, researchers often end up wasting time, effort and research funds when following up on false leads, mainly because of ambiguity in the methodology used. This often leads to unnecessary delays in research, and even worse, can lead to incorrect or conflicting results that are not publishable. Moreover, it erodes public trust in scientific research.
In a 2014 white paper, the American Society for Cell Biology (ASCB) provided a multi-tier explanation of reproducibility in science, explaining the different types of replicability in research.3
- Direct Replication: This is when the original experiments are replicated using the same materials, methods, and conditions to get the previously observed results.
- Conceptual Replication: This is when the validity of a concept is evaluated using a different paradigm or situation.
- Systematic Replication: This refers to the use of different experimental conditions to replicate the results in a previously published study.
- Analytic Replication: This is the attempt to reanalyze the original data shared in order to reproduce the same scientific results
Challenges with reproducibility and replicability in research
While a basic expectation from an author is the reproducibility in science, it is not always the case. Several research studies, especially in biomedical research, present significant findings that cannot be replicated. This was proven by a Nature survey in 2016 that showed more than 70% of researchers in the field of biology were unable to replicate the findings of other scientists, and approximately 60% researchers were unable to reproduce their own results.1 This may raise doubts about the efficacy of the research hypothesis and the various methods used to come up with the results.
Reproducibility and replicability in research are issues that many institutes and funding agencies are keen to resolve. Failures in scientific reproducibility, however, cannot be attributed to any one reason. Take a look at the key factors affecting reproducibility in science.
Reproducibility and replicability in research: Challenges and way ahead1
1. Difficulties dealing with complex data
A lack of understanding on the part of researchers on how to manage, analyze and store complex data correctly can lead to problems with replicability in research. Moreover, advances in technology and newer methodologies are not standardized, which could introduce biases and variations that result in misinterpretations or incorrect results.
To mitigate these problems, researchers should be trained, not only to structure experiments and analyse results effectively, but also to strictly follow best practices in statistical methods and study design. This increased awareness and training can improve authenticity and validity of researchers’ statistical data and boost the chances of scientific replicability in research.
2. Limited access to research materials, methodologies, or raw data
In order to ensure reproducibility of results, academics need access to original data, methods, and key research materials. However, this is often not the case and researchers are forced to start from scratch and figure out how to get the previous results.
In order to ensure reproducibility in science and research, data sharing systems should be made openly and freely available. One way to do this would be to share raw data from previously published work in a public database so that researchers can select results that support or confirm existing concepts or results. This would help reduce confusion, cut down on researcher effort, encourage collaboration and speed up the pace of reliable, replicable scientific discoveries.
3. Use of invalidated or contaminated biomaterials
Reproducibility in science can be affected by the use of biomaterials that cannot be traced back to the original source, are not verified, or have not been stored properly. The use of contaminated or misidentified materials can also lead to questionable or invalid results, which have issues with scientific reproducibility of research.
One way to overcome this challenge is by ensuring data integrity with the use of biomaterials that are verified and can be traced back to the original source. It is also important to ensure the use uncontaminated materials that is evaluated frequently for quality in order to create reliable research results that are also likely to be replicable and reproducible.
4. Poor research practices and incorrect experimental design
While working in the laboratory, a lack of clear experimental parameters or poorly designed studies, where the methodology is not reported correctly, has a big impact on reproducibility in research. It is critical for scholars to pay attention to how they plan and design their laboratory experiments, which should be based on an in-depth, unbiased study of existing evidence.
In order to ensure reproducibility and replicability in research, it is crucial for researchers to clearly note and report key experimental parameters, including the kinds of tools, equipment, and standards used, to explain how statistical analysis was conducted, and how data was interpreted. The detailed and accurate description of the research methodology used to come up with previously published results can also help other researchers replicate the experiments effectively, which helps boost reproducibility in science and research.
5. Rewarding positive findings but ignoring negative results
In a highly competitive research landscape, the tendency is to reward and recognize novel findings that prove a hypothesis instead of research with negative results. This competitiveness also limits the extent of data or details researchers share about learnings through experiments that do not work. The rampant under-reporting of research with seemingly insignificant results can cost researchers the loss of precious time, effort and money and further hamper reproducibility and replicability in research.4
There is a need for more platforms and better awareness of the need to publish negative results, which could help researchers avoid duplicate efforts in trying to replicate irreproducible research. Further support by institutes, funders, and publishers to promote and publish research with negative data that is not considered as innovative or high impact could help academics interpret results better and adjust their experimental design to optimize the use of resources and efforts.
In the scientific publishing sphere, it is critical to understand that bad science only works to undercut overall trust in research but also impacts a researcher’s credibility. While researchers are expected to ensure replicability in research results, top funders and publishers also need to work toward highlighting issues with reproducibility in research and encourage better practices among scientists.
References
- Six factors affecting reproducibility in life science research and how to handle them. Nature Portfolio. Retrieved from https://www.nature.com/articles/d42473-019-00004-y#ref-CR1
- Resnik D.B., Shamoo A.E. Reproducibility and Research Integrity. PubMed Central, November 2016. https://www.ncbi.nlm.nih.gov/pmc/articles/PMC5244822/
- Walter P. On Reproducibility and Clocks. President’s Column, ASCB, April 2016. https://www.ascb.org/publications-columns/presidents-column/on-reproducibility-and-clocks/
- Nimpf S., Keays D.A. Why (and how) we should publish negative data. EMBO Reports, January 2020. PubMed Central https://www.ncbi.nlm.nih.gov/pmc/articles/PMC6945059/
R Discovery is a literature search and research reading platform that accelerates your research discovery journey by keeping you updated on the latest, most relevant scholarly content. With 250M+ research articles sourced from trusted aggregators like CrossRef, Unpaywall, PubMed, PubMed Central, Open Alex and top publishing houses like Springer Nature, JAMA, IOP, Taylor & Francis, NEJM, BMJ, Karger, SAGE, Emerald Publishing and more, R Discovery puts a world of research at your fingertips.
Try R Discovery Prime FREE for 1 week or upgrade at just US$72 a year to access premium features that let you listen to research on the go, read in your language, collaborate with peers, auto sync with reference managers, and much more. Choose a simpler, smarter way to find and read research – Download the app and start your free 7-day trial today!