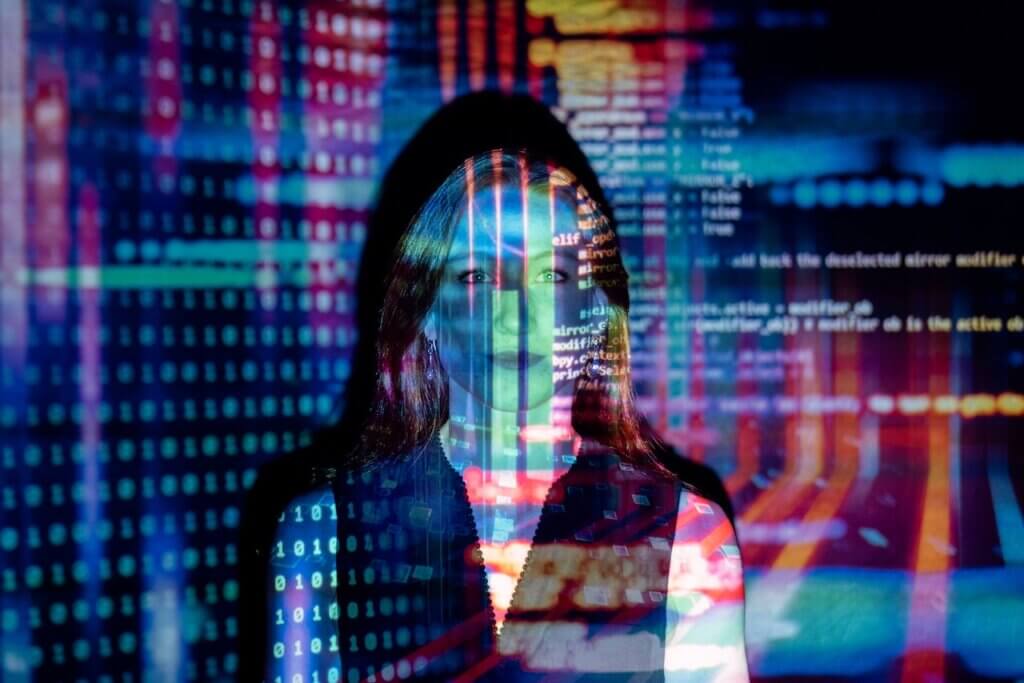
Our society is awash in data. We have become extremely adept at collecting and storing amazing amounts of information and navigating through that data to find patterns and associations, or knowledge1. This ability has launched a new discipline known as “big data.” Although the term big data is nearly ubiquitous these days and appears in all areas of life, there is not a single agreed upon definition. So, what makes using big data in scientific research different from using traditional data?
The differences between traditional and big data lie in the latter’s 5V characteristics: huge volume, high velocity, high variety, low veracity, and high value1. The massive amounts of available data include both quantitative and qualitative information and are obtained from both the physical world and from human society. Although volume is the most obvious characteristic, data types (variety) and data uncertainties (veracity) also have significant impacts on the use of big data in scientific research.
Big data’s impact on scientific research
The development of big data in science and research has initiated changes in the basic nature of research and the scientific method. In a traditional research study, a hypothesis is proposed, data is collected and analyzed, and conclusions based on the original hypothesis are reached. However, with big data, large datasets are mined, patterns and associations are found, and then hypotheses are proposed and tested1.
In this revised research method, simulations are negating the need for physical experiments, and some researchers never actually conduct physical experiments. Big data in medical research is a major example of this. For instance, genome sequencing technology provides researchers with the ability to study human traits and diseases through massive data on individuals2; thus, big data offers the potential to improve the quality of life for millions of people.
The diverse sources of data currently being collected, including images, text, and audio, make more complete information available on many topics. These large and rich datasets allow researchers the opportunity to deepen their understanding of their chosen discipline, be that neurology, astronomy, or marketing2.
Challenges of using big data in research
However, while big data offers unprecedented opportunities, it also presents major challenges to researchers who use big data in scientific research.
One of the biggest challenges presented by big data involves data uncertainty. Big data can also mean less reliable data. So much information is collected from so many diverse sources, it’s difficult to know which to trust. In addition, researchers, or other team members, must spend a great deal of time and effort cleaning the data before analyzing it or risk reaching inaccurate conclusions. A certain amount of expertise is required to do this effectively.
The massive volume and diversified types of data involved also make data analysis more difficult. Not only are more resources and advanced tools needed to support the analysis but a good understanding of how to handle the increased complexity of big data is currently lacking1,2. New methods of causal inference and new models and experimental designs must be developed to take full advantage of the available information, which will involve updated assumptions, more complex iterative algorithms, and more advanced statistical approaches.
Advancement in the technological support to handle the analysis complexity will also be crucial. The development of energy-efficient big data platforms is a key issue along with new system architecture designs and processing modes1.
Tips for researchers on using big data in science and research
- Understand your data: It is essential that researchers know everything about the data they are using, such as where and how it was collected, including sampling method, the context, and any limitations. While this has always been important, the use of big data has increased the divide between researchers and their data. Know what your data can and cannot tell you.
- Visualize your data: One good way to help you become familiar with your data is to use visualizations3. By graphing the data, information on outliers or other bad behavior can be found before those datapoints are included in the analysis.
- Document everything: Big data studies are notoriously difficult to replicate. Make it easy for anyone trying to validate your work by taking notes on everything you do3. Make those notes and the data available to your teammates. Showing your work is still as important as it was when you were struggling through algebra.
- Be a skeptic: Be careful to validate your conclusions to avoid generalizing a piece of knowledge found in a large dataset that may be only a small coincidence.
- Learn to program: Take a course in R, Python, or whatever your group or institution is using3. Even if you are not doing the programming, it always helps to speak the language.
- Ask for help: Finally, you do not have to be an expert in everything. Research is not a solo act.
References
- Jin, X. et al. Significance and challenges of big data research. Big Data Research (2015). http://dx.doi.org/101016/j.bdr.2015.01.006
- Blei, D. M., Smyth P. Science and data science. PNAS 14, 8689–92 (2017). https://doi.org/10.1073/pnas.1702076114
- Nowogrodzki A. Eleven tips for working with large data sets. Nature 577, 439–440 (2020). doi: https://doi.org/10.1038/d41586-020-00062-z