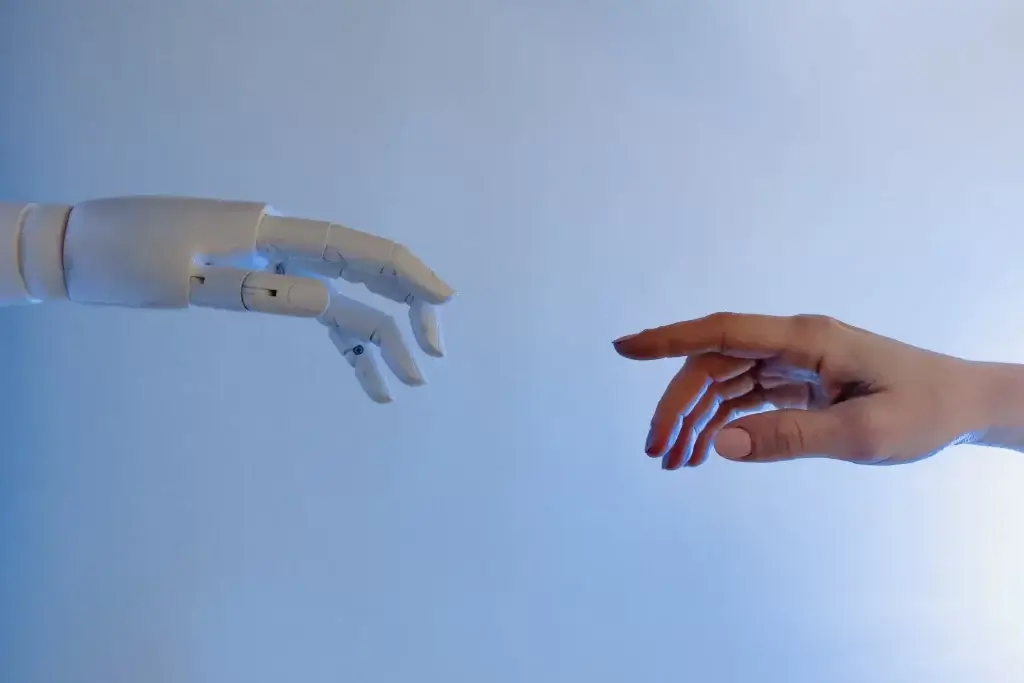
With automation taking center stage in most industries, one cannot help but wonder the impact AI in publishing can bring. With AI, there are umpteen possibilities of applying artificial intelligence (AI) and machine learning in publishing research. However, the use of tools powered by AI in research and the scholarly publishing industry is a double-edged sword as there is still debate in the scientific community regarding its ethical as well as social implications. One of the biggest concerns is the development of confirmation bias that might occur while using tools driven by deep-learning algorithms, which are programmed to generate repeated patterns in an existing data set by “thinking like humans.”1 While this is a valid concern, it can be tackled effectively by undertaking necessary precautions when using AI in publishing and research, as discussed briefly toward the end of this post.
Regardless of the diversity in opinions, the role of AI and machine learning in research will continue to gain precedence in the near future. Let’s take a look at some of the distinctive features of the commonly used tools driven by AI in publishing and research:
- Literature-discovery tools: As a researcher, you might already be aware of the tedious nature of the process involved in searching for and managing relevant literature. Artificial intelligence for publishers and researchers is making that easy. Artificial intelligence and machine learning is continuously bringing significant changes in research and in the process of how you search for relevant literature. Further, the search can be personalized by taking into consideration your specific interests and making relevant suggestions. One example of such a tool is R Discovery, which uses a large repository of scientific literature, learns user preferences, and recommends recent/relevant papers on a daily basis.
- Article-summarizer tools: We are living in an era where knowledge needs to be dissipated quickly, and “too long didn’t read” has become quite the norm. AI in publishing and research has solved this problem to an extent with tools that generate a quick summary of articles. Article-summarizer tools are specifically programmed to capture relevant keywords and highlights from the article text. Notable among these AI tools in research are TLDR This, Scholarcy, and UNSILO. If you are keen to conserve the time spent on literature review, then these tools which use machine learning in publishing and research, can be extremely useful for you.
- Tools for identifying plagiarism: Spotting plagiarism in submitted manuscripts can become tiring for publication houses, especially with the rapid increase in number as well as the frequency of submissions every year. AI in publishing and research has changed the game completely. With AI-driven tools for researchers such as Copyleaks and Proofig, it has become easier to spot plagiarism in text and images and uphold scientific integrity.
- Language-editing and proofreading tools: One of the most popular applications of machine learning in publishing and research has been the development of language-support tools. Language-editing tools such as Paperpal are extremely useful in identifying grammatical errors and ensuring accurate usage of domain-specific technical terminology. Such tools driven by AI in research offer both authors and journal editors substantial advantages. Authors can perform a pre–peer preview check on their manuscripts to enhance the quality of their manuscripts. On the other hand, journal editors can focus on evaluating the content of the submitted manuscript for novelty and alignment with journal scope rather than struggle to understand the content because of poor language quality. With the growing use of AI in publishing research, the turnover time in an average publication cycle can be reduced by a significant amount of time.
Another application of AI in publishing research is that of finding relevant reviewers in the peer-review process. Tools that help with this will not only speed up the search but also enhance the process of identifying reviewers who have previous experience in a specified research field. In an era of collaborative multi-author and multi-institutional publications, such AI-driven tools can also help identify whether the selected reviewers have any conflicts of interest.2
The use of artificial intelligence for publishers and researchers is surely going to change the landscape of scholarly publications as we know it. However, in order to tackle the concerns described earlier in the article, it is essential to avoid complete reliance on the results generated by machine-learning algorithms. For example, human oversight and judgment are critical before making any final decisions regarding acceptance/rejection of manuscripts or ethical misconduct. Machine-learning algorithms in research and literature-discovery tools should be updated periodically, in order to avoid repeated pattern generation.3
Thus, one can safely assume that despite the developments related to AI in publishing and in research, human role will continue to have its own place in academic publishing. Artificial intelligence for publishers and researchers can help, but human judgement can never be replaced.
References
- González-Esteban y Patrici Calvo, E. Ethically governing artificial intelligence in the field of scientific research and innovation. Heliyon 8, e08946 (2022).
- This is how AI will speed up peer reviews. Editage Insights https://www.editage.com/insights/this-is-how-ai-will-speed-up-peer-reviews (2020).
- What you need to know about artificial intelligence in research and publishing. Editage Insights https://www.editage.com/insights/what-you-need-to-know-about-artificial-intelligence-in-research-and-publishing (2022).